
In today’s fast-paced business environment, unlocking data to make informed decisions is more crucial than ever. Data Analytics and Business Intelligence (BI) have emerged as powerful tools that unlock raw data into actionable insights. Companies that effectively unlock these tools can gain a competitive edge, unlock operations, and better understand their customers. This comprehensive blog will explore the key concepts, benefits, tools, and future trends in Data Analytics and Business Intelligence, providing a thorough understanding of how these disciplines can unlock businesses.
Introduction to Data Analytics and Business Intelligence
What is Data Analytics?
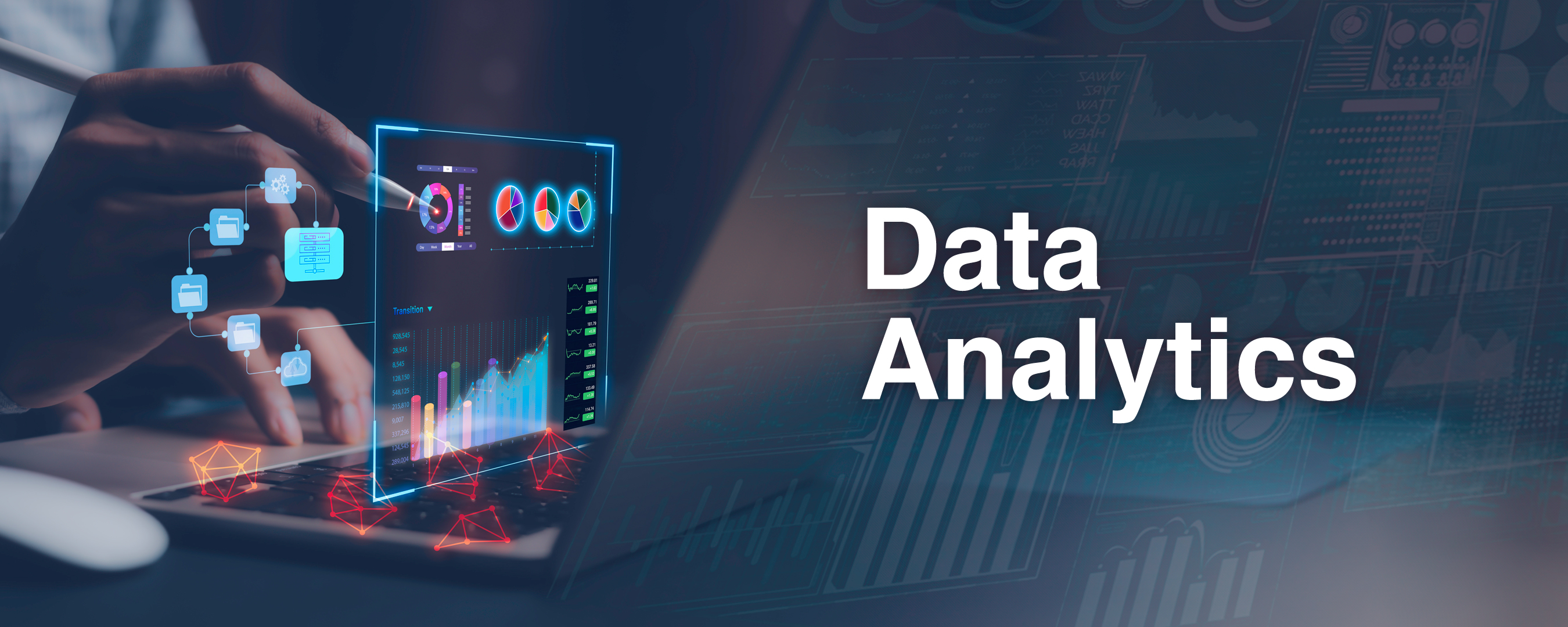
Data Analytics involves examining raw data to uncover patterns, trends, and insights. Initially, it employs statistical and computational techniques to analyze data sets. Subsequently, meaningful conclusions are derived. Furthermore, the process begins with data collection. Next, it moves on to data cleaning and transformation. Ultimately, it culminates in data modeling. The process of Data Analytics includes several stages such as data collection, data cleaning, data transformation, and data modeling.
What is Business Intelligence?
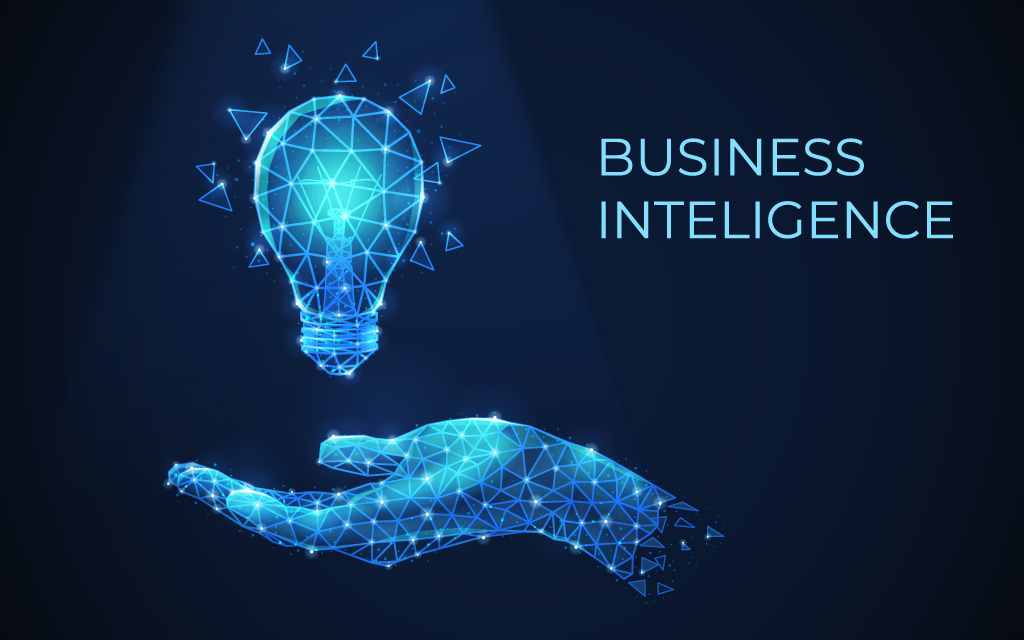
Business Intelligence, in essence, refers to technologies, applications, and practices for the collection, integration, analysis, and presentation of business information. First, the goal of BI is to support better business decision-making. Moreover, BI systems are essentially data-driven Decision Support Systems (DSS). Therefore, by leveraging these systems, organizations can derive meaningful insights. Consequently, this leads to more informed strategic choices. Finally, the integration of BI into daily operations significantly enhances overall business performance.
How Do They Differ and Interrelate?
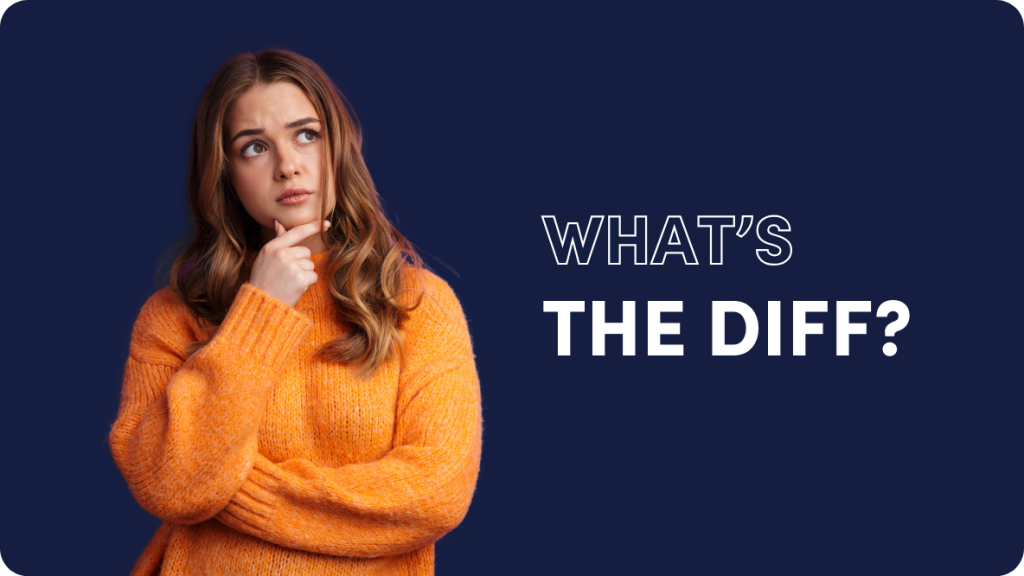
While Data Analytics and Business Intelligence are often used interchangeably, they are distinct but complementary fields. Data Analytics is more focused on statistical analysis and predictive modeling, whereas BI is more concerned with the collection, integration, and visualization of business data to inform decision-making. Together, they provide a comprehensive framework for understanding and leveraging data within an organization.
The Importance of Data Analytics and Business Intelligence
Enhancing Decision-Making
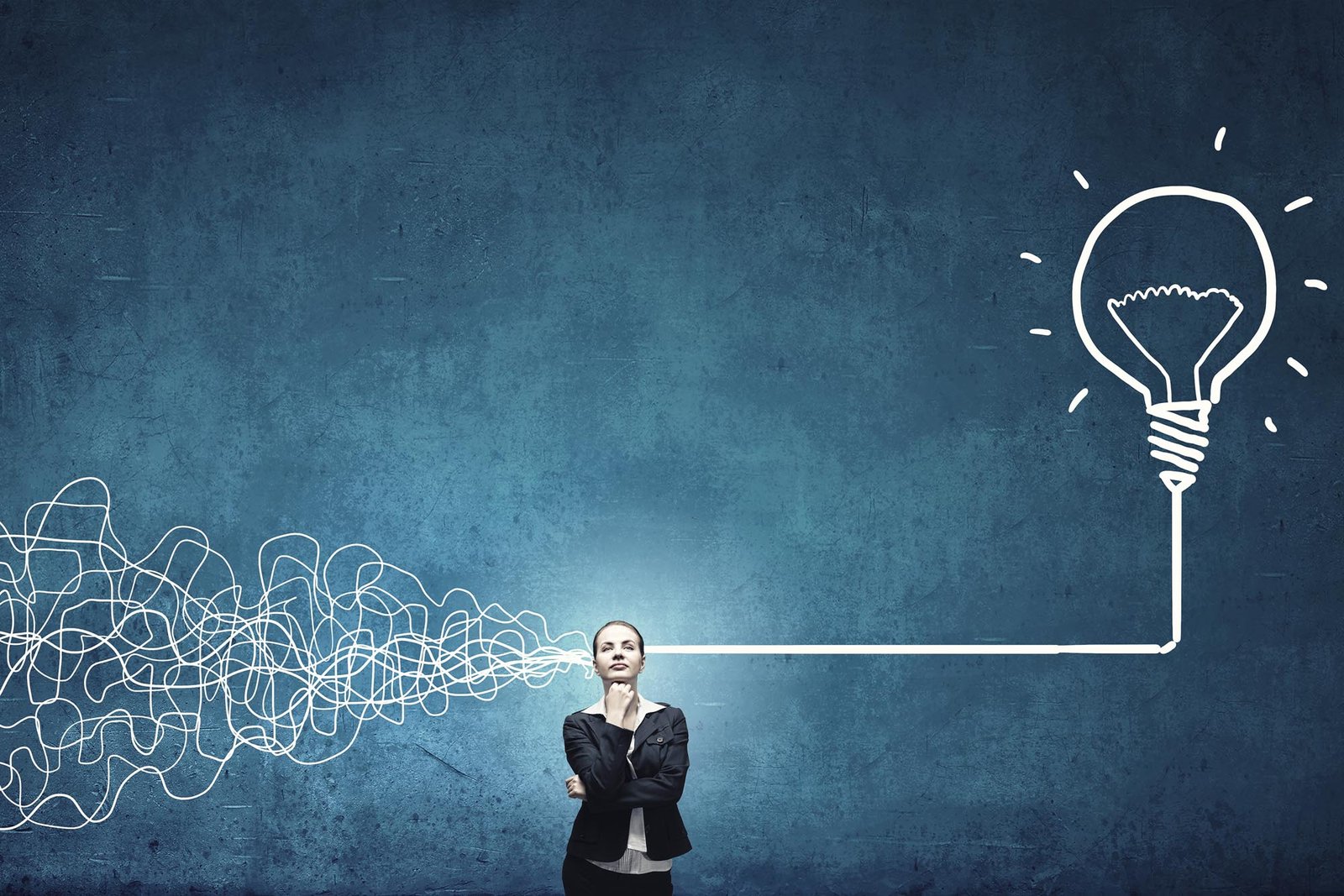
Unlocking the potential of Data Analytics and BI entails empowering enhanced decision-making. By unlocking real-time data and insights, businesses can make more informed decisions, reducing reliance on gut feelings or guesswork and ensuring decisions are based on solid evidence.
Improving Operational Efficiency
Data Analytics and BI can significantly improve operational efficiency. By analyzing data from various business processes, organizations can identify bottlenecks, streamline operations, and reduce costs. For example, predictive analytics can forecast demand, allowing companies to optimize inventory levels and avoid overstocking or stockouts.
Gaining Competitive Advantage
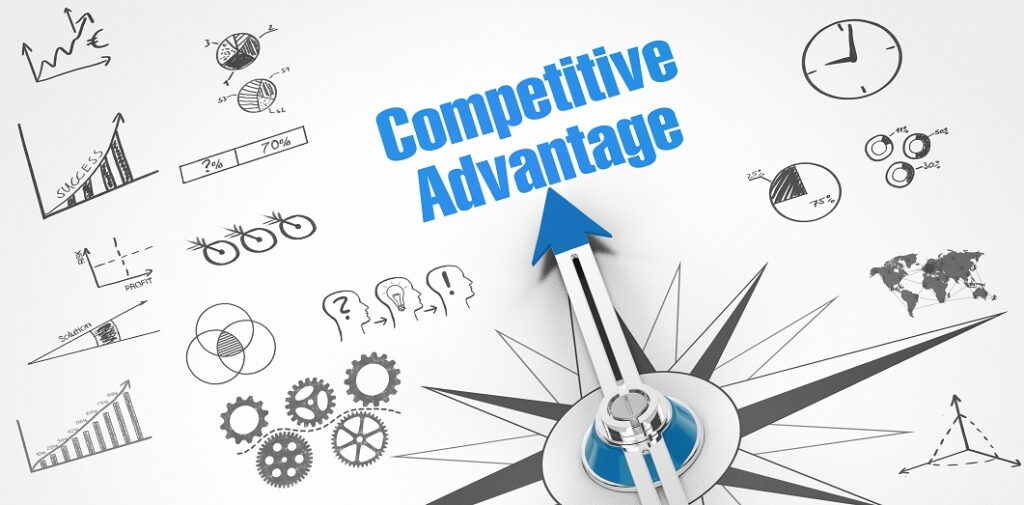
In today’s competitive market, having a data-driven approach can provide a significant advantage. Companies that leverage Data Analytics and BI can better understand market trends, customer preferences, and competitor actions. This insight enables them to respond more quickly to market changes and stay ahead of the competition.
Enhancing Customer Experience
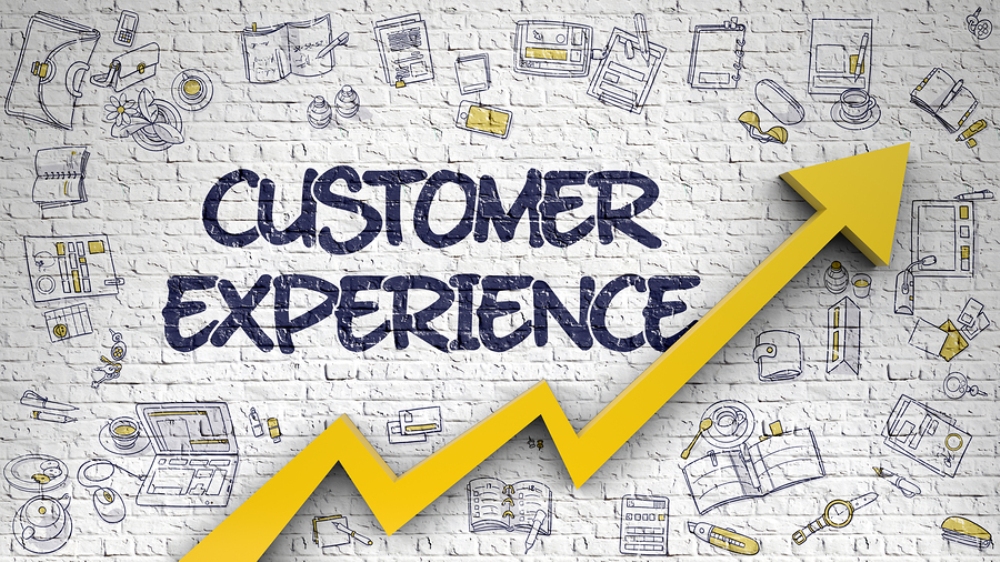
Understanding customer behavior and preferences is key to delivering a superior customer experience. Data Analytics and BI tools can analyze customer data to identify patterns and trends. Consequently, businesses can personalize their offerings and, therefore, improve customer satisfaction.
Facilitating Strategic Planning
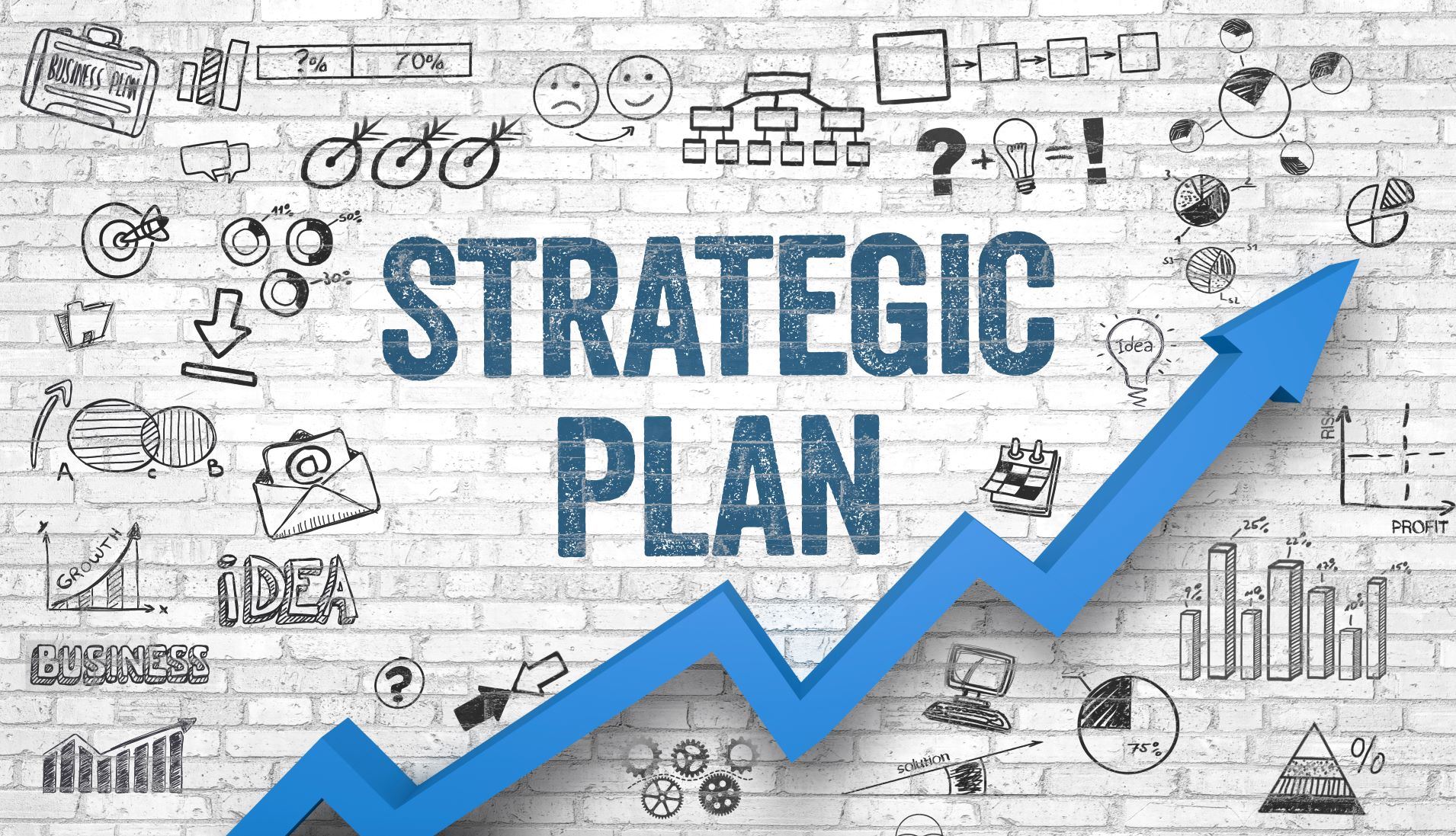
Strategic planning is critical for long-term success, and Data Analytics and BI play a crucial role in this process. By providing a comprehensive view of the business landscape, these tools enable organizations to develop more effective strategies and achieve their long-term goals.
Key Concepts in Data Analytics and Business Intelligence
Descriptive Analytics
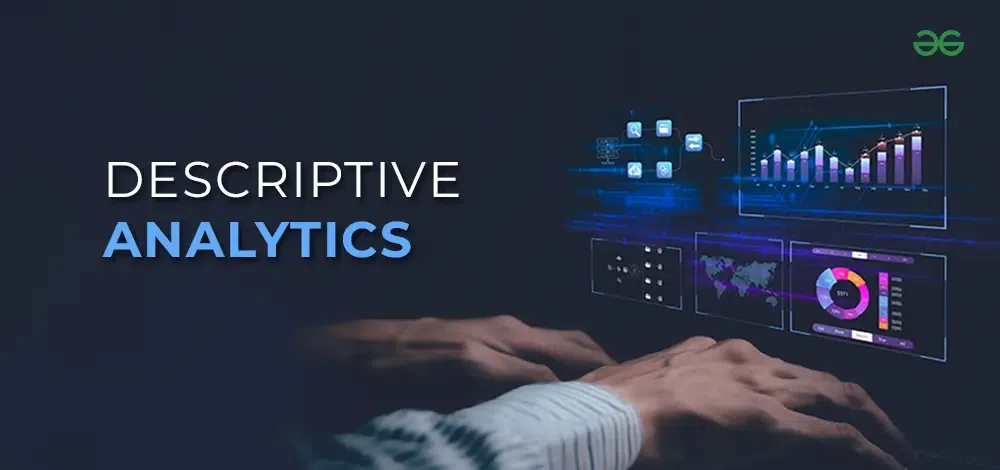
Descriptive Analytics focuses on summarizing historical data to understand what has happened in the past. It involves techniques such as data aggregation and data mining to provide insights into past performance.
Predictive Analytics

Predictive Analytics uses statistical models and machine learning techniques to forecast future outcomes based on historical data. It helps businesses anticipate trends, identify risks, and make proactive decisions.
Prescriptive Analytics

Prescriptive Analytics goes a step further by recommending specific actions to achieve desired outcomes. It combines data, algorithms, and business rules to suggest optimal decisions and actions.
Data Mining

Data Mining involves exploring and analyzing large data sets to discover patterns and relationships. It employs techniques such as clustering, classification, and association rule learning to uncover hidden insights.
Data Visualization

Data Visualization is the graphical representation of data and information. It involves creating charts, graphs, and dashboards that make complex data more accessible and understandable. Effective visualization helps stakeholders quickly grasp insights and trends.
Big Data

Big Data refers to the vast volumes of structured and unstructured data generated by businesses and individuals. Managing and analyzing Big Data requires specialized tools and techniques due to its size, complexity, and speed of generation.
Machine Learning
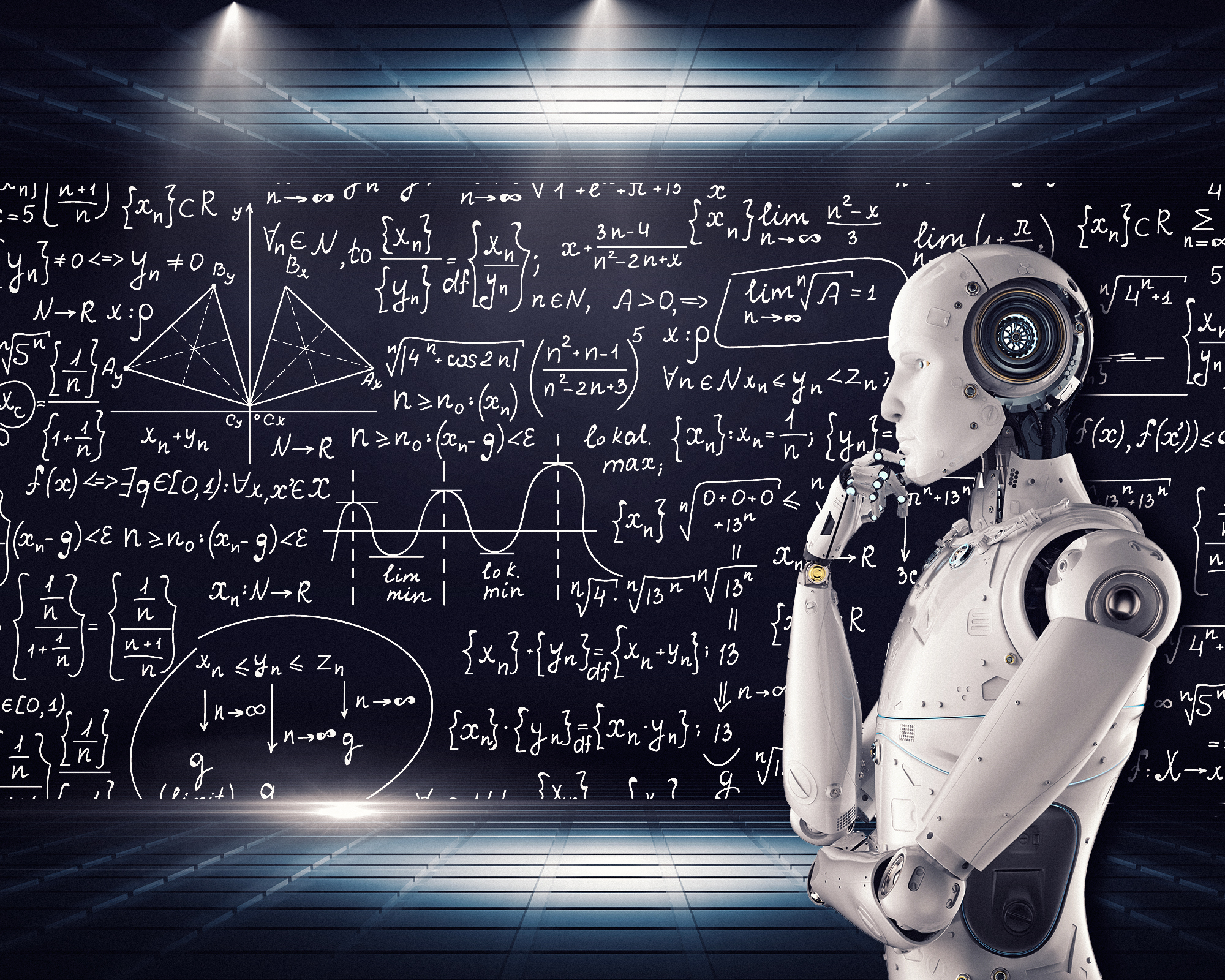
Machine Learning is a subset of artificial intelligence that involves training algorithms to learn from data and make predictions or decisions. It plays a crucial role in predictive analytics and other advanced analytics applications.
Tools and Technologies in Data Analytics and Business Intelligence
Data Warehousing
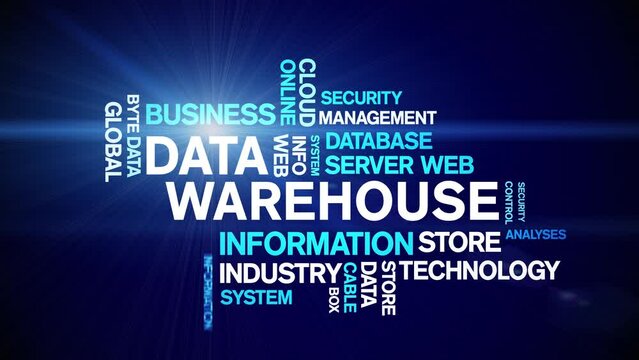
A Data Warehouse, acting as a centralized repository, accumulates large volumes of data from diverse sources. Consequently, it facilitates data analysis and reporting by offering a unified view of the data. Notably, popular data warehousing solutions like Amazon Redshift, Google BigQuery, and Snowflake exemplify this approach.
ETL (Extract, Transform, Load)
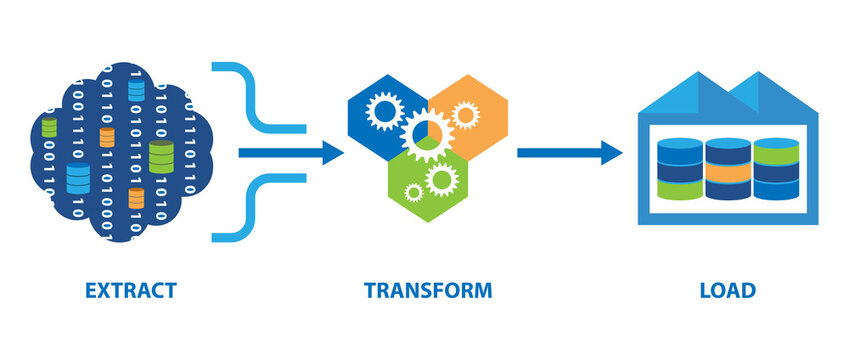
The ETL process is about unlocking data from diverse sources, transforming it into a compatible format, and unlocking its potential by loading it into storage systems like data warehouses. Apache Nifi, Talend, and Informatica are widely used tools for unlocking data in ETL processes.
Business Intelligence Platforms

BI platforms unlock a range of tools for data analysis, reporting, and visualization. They empower users to create interactive dashboards and reports, unlocking insights into business performance. Leading BI platforms, such as Microsoft Power BI, Tableau, and QlikView, further unlock the potential for enhanced decision-making.
Data Analytics Tools
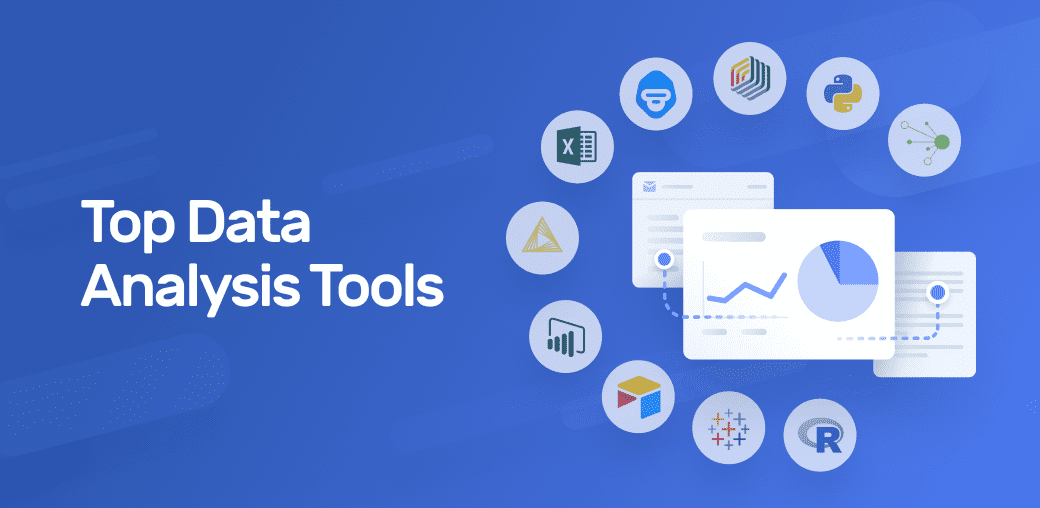
Unlock the potential of Data Analytics tools, harnessing advanced analytical capabilities such as statistical analysis, machine learning, and data mining. Among the popular tools in this category are Apache Spark, R, and Python libraries like pandas and scikit-learn, unlocking a myriad of possibilities for data exploration and insight generation.
Cloud Computing
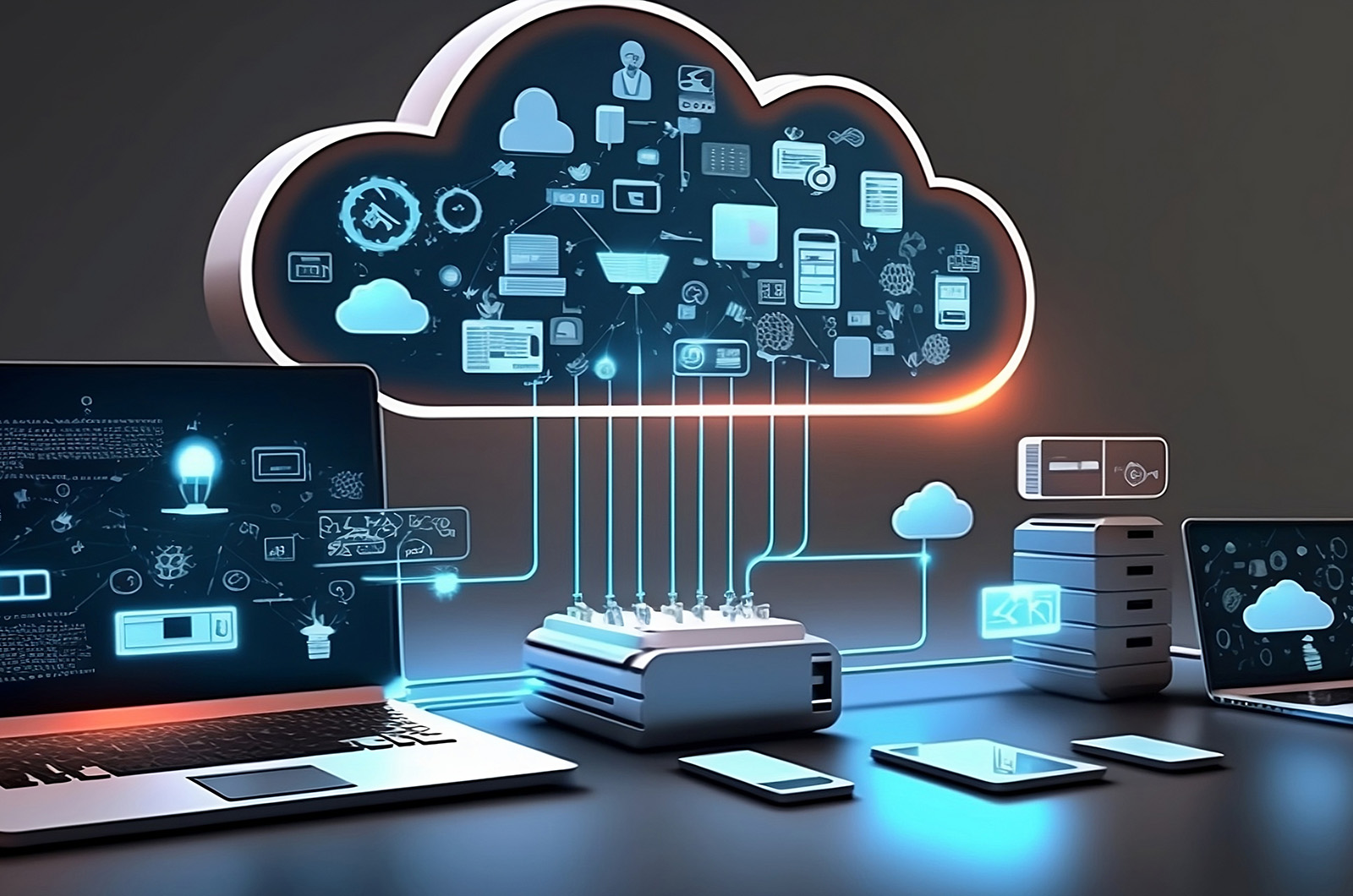
It has revolutionized Data Analytics and BI by providing scalable and cost-effective solutions. Cloud platforms such as Amazon Web Services (AWS), Microsoft Azure, and Google Cloud Platform offer a wide range of data services, including data storage, analytics, and machine learning.
Artificial Intelligence and Machine Learning Platforms
AI and Machine Learning platforms provide tools and frameworks for developing, training, and deploying machine learning models. Examples include TensorFlow, PyTorch, and IBM Watson.
Data Integration Tools
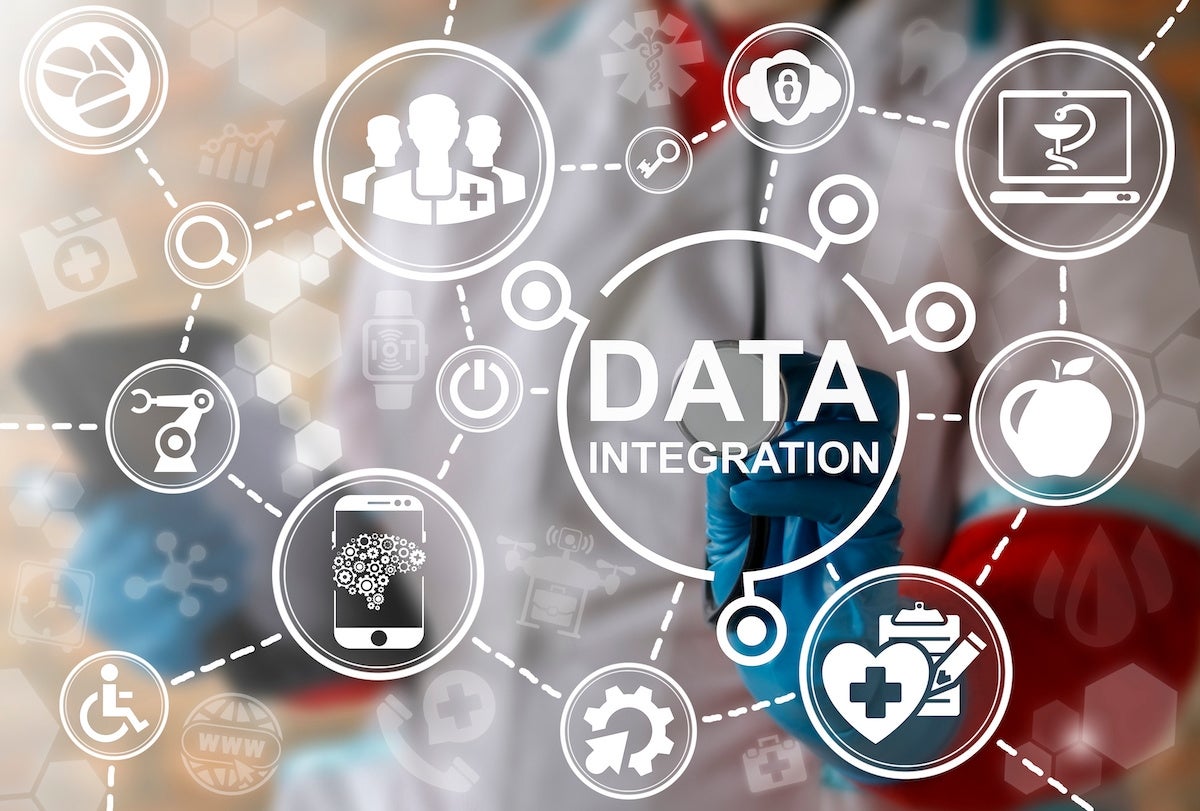
Data Integration tools facilitate the process of combining data from different sources into a unified view. They support various data formats and provide capabilities for data cleaning, transformation, and loading. Examples include Apache Kafka, MuleSoft, and Microsoft SQL Server Integration Services (SSIS).
Implementing Data Analytics and Business Intelligence
Unlock Defining Objectives and Goals
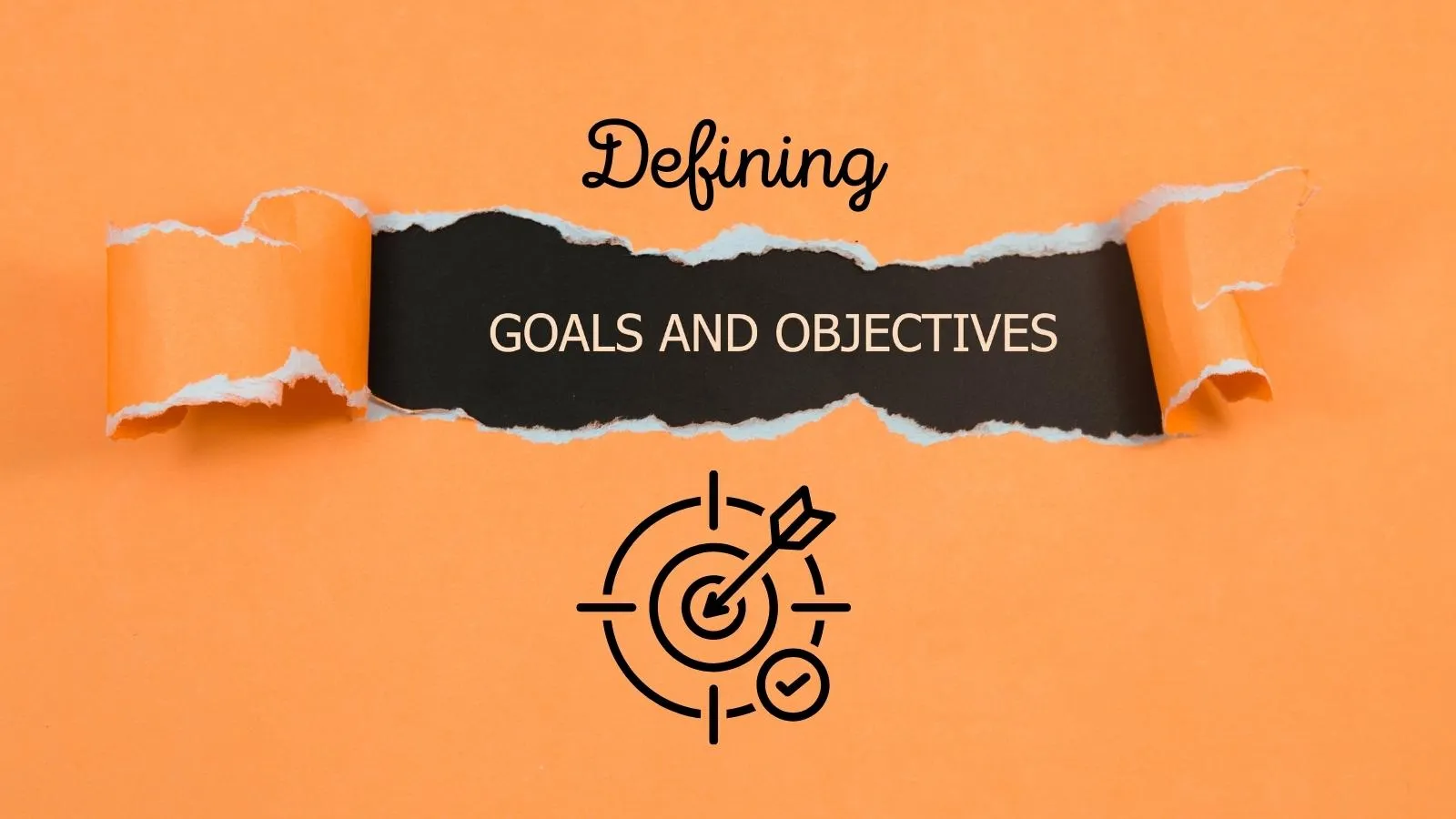
Unlocking the potential of Data Analytics and BI begins with defining clear objectives and goals. Unveiling what you want to achieve will unlock the selection of tools, techniques, and metrics. Whether it’s enhancing sales performance, streamlining operations, or enriching customer experience, unlocking well-defined goals is paramount.
Unlock Data Collection and Management
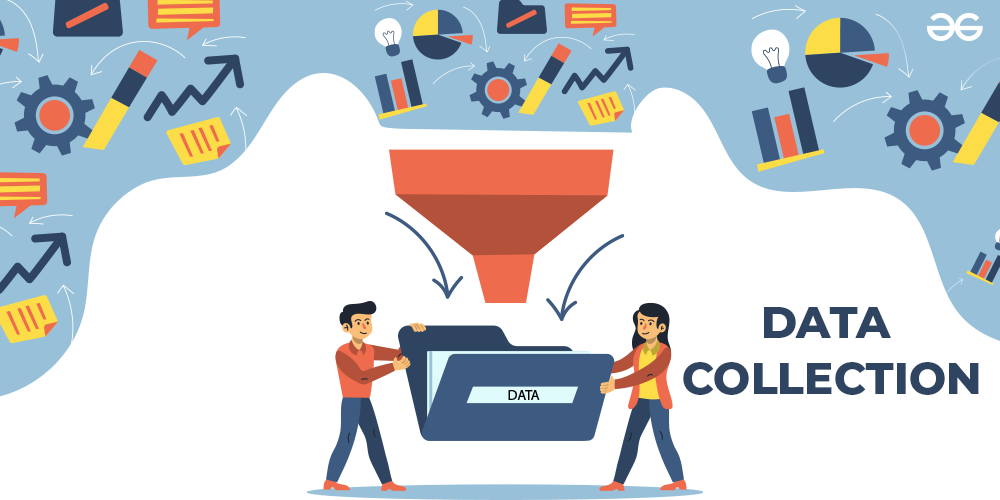
Unlocking the potential of data begins with collecting and managing it effectively. This entails identifying relevant data sources, ensuring its quality, and establishing robust data governance practices. By unlocking data, organizations guarantee its accuracy, consistency, and accessibility, laying the foundation for informed decision-making and strategic insights.
Unlock Selecting the Right Tools

Unlocking the ideal tools for Data Analytics and BI hinges on your organization’s unique requirements and capacities. Factor in elements like user-friendliness, scalability, integration potential, and cost to unlock the best fit. Ensure these tools can effectively unlock the volume and complexity of your data.
Unlock Building a Skilled Team
For a successful Data Analytics and BI implementation, assembling a skilled team is crucial. Specifically, this team should consist of data analysts, data engineers, data scientists, and BI developers. Together, they can collaborate effectively to achieve your goals.
Unlock Data Analysis and Visualization
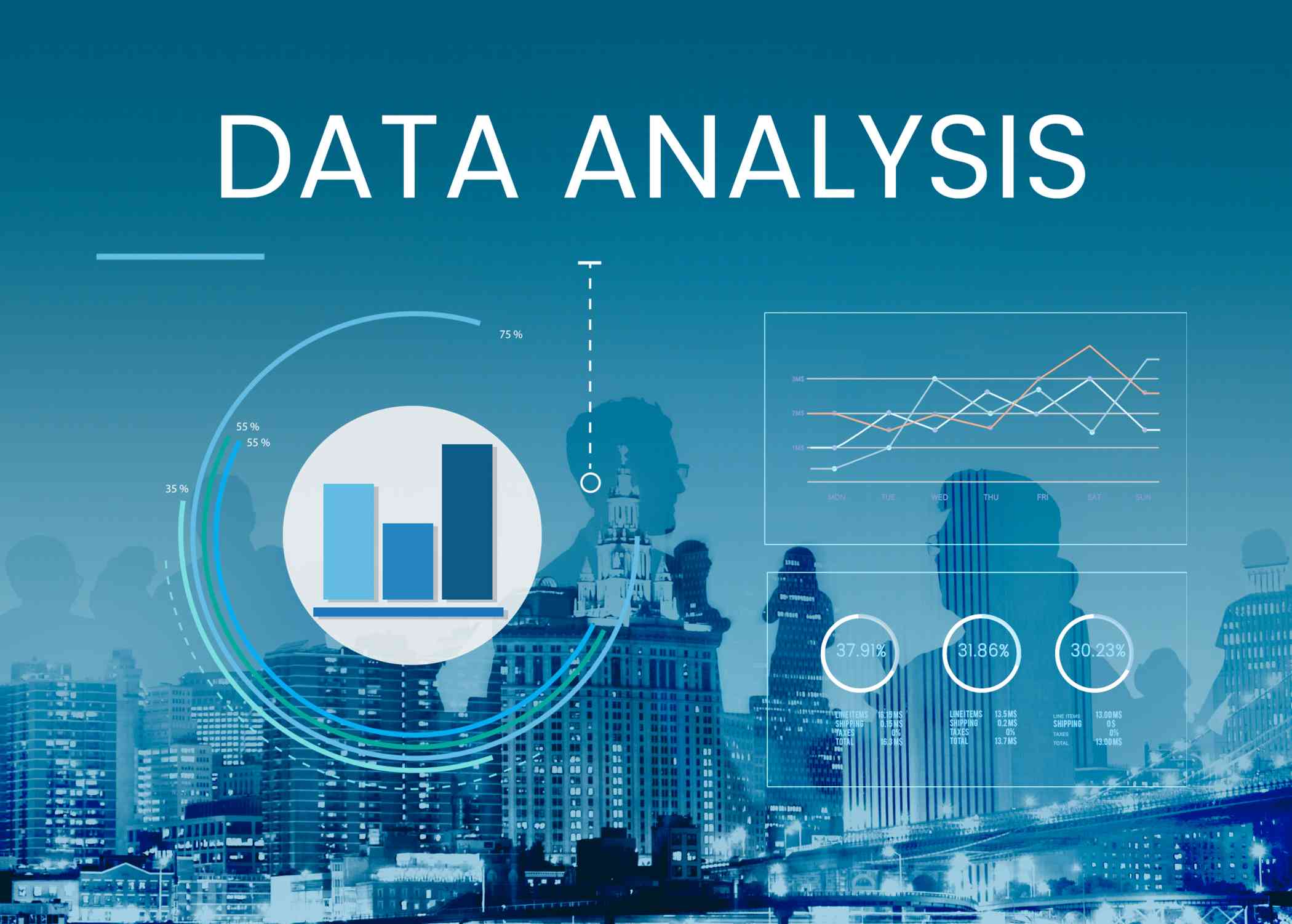
Once the data is collected and managed, the next step is to analyze it and create visualizations. This involves applying statistical techniques, machine learning algorithms, and data mining methods to uncover insights. Effective visualization is key to communicating these insights to stakeholders.
Unlock Reporting and Actionable Insights
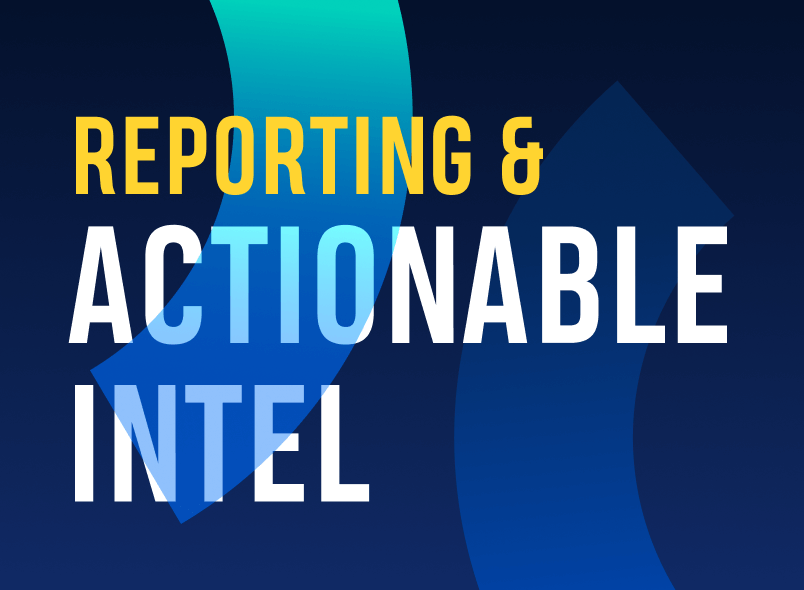
The ultimate step entails generating reports and providing actionable insights to decision-makers. Subsequently, this involves creating dashboards, reports, and presentations that highlight key findings and recommendations. Crucially, ensuring that these insights are actionable and aligned with business objectives is pivotal for driving impact.
Challenges in Data Analytics and Business Intelligence
Unlock Data Quality Issues
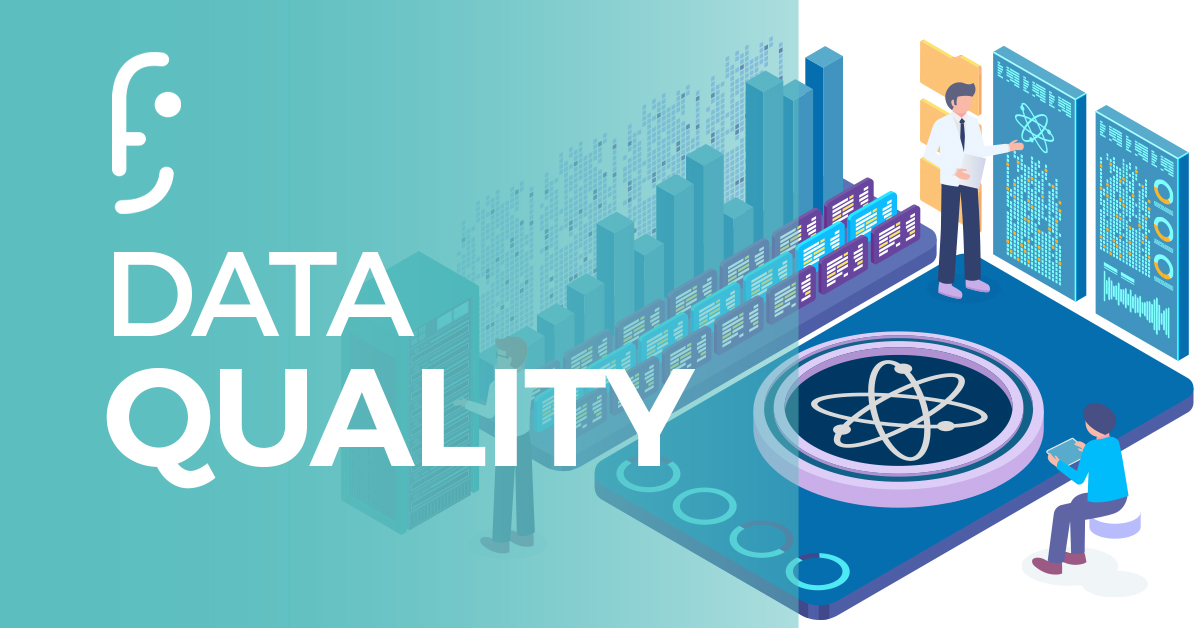
Data quality presents a significant challenge in Data Analytics and BI. Consequently, inaccurate, incomplete, or inconsistent data can lead to misleading insights and poor decision-making. Therefore, implementing data quality management practices is essential to ensure the reliability of your data.
Unlock Data Security and Privacy
With the increasing volume of data, ensuring data security and privacy is more important than ever. Businesses must implement robust security measures to protect sensitive information and comply with data protection regulations such as GDPR and CCPA.
Unlock Integration of Diverse Data Sources

Integrating data from diverse sources can be complex and time-consuming. Moreover, ensuring that data from different systems and formats can be seamlessly integrated and analyzed is a significant challenge. Nevertheless, utilizing data integration tools and establishing standardized data formats can help address this issue. Consequently, businesses can streamline their data processes. Additionally, this enables more accurate and efficient data analysis. Ultimately, such strategies lead to better decision-making and improved operational efficiency.
Keeping Up with Technological Advancements
The field of Data Analytics and BI is constantly evolving. Moreover, new tools, technologies, and methodologies emerge regularly. Therefore, keeping up with these advancements is essential. Additionally, continuously upgrading your skills and tools is crucial. Consequently, staying competitive requires constant learning. Furthermore, embracing innovation ensures you remain at the forefront of the industry.
Unlock Talent Shortage
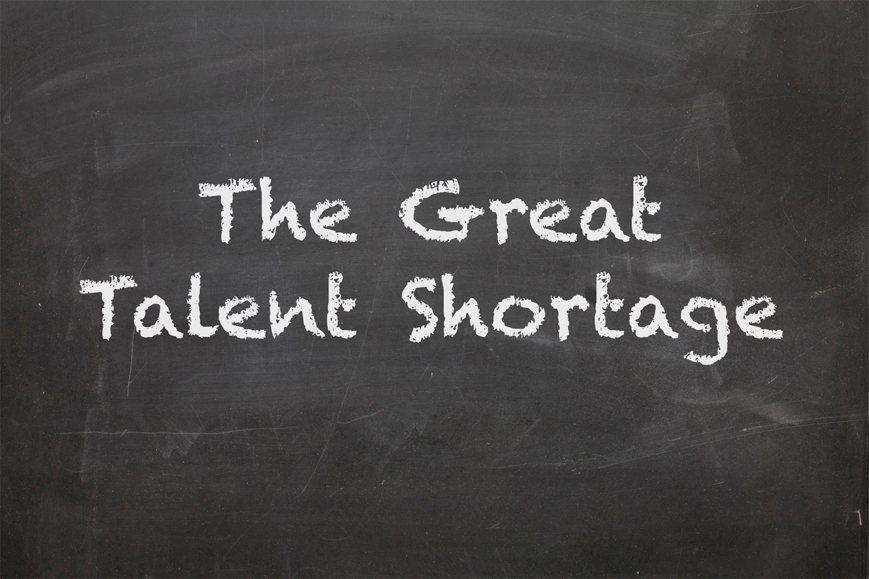
There is a high demand for skilled professionals in Data Analytics and BI, leading to a talent shortage in the industry. To address this challenge, first, building a skilled team is crucial. Additionally, investing in training programs is essential. Finally, ongoing development initiatives can ensure continuous improvement.
Future Trends in Data Analytics and Business Intelligence
Unlock Artificial Intelligence and Machine Learning
AI and Machine Learning are set to play an even more significant role in Data Analytics and BI. These technologies can automate complex tasks, provide deeper insights, and enable more accurate predictions. As AI and ML continue to evolve, consequently, their integration into BI platforms will become more seamless. Furthermore, this integration will become more widespread, ultimately enhancing the capabilities of business intelligence systems.
Unlock Augmented Analytics
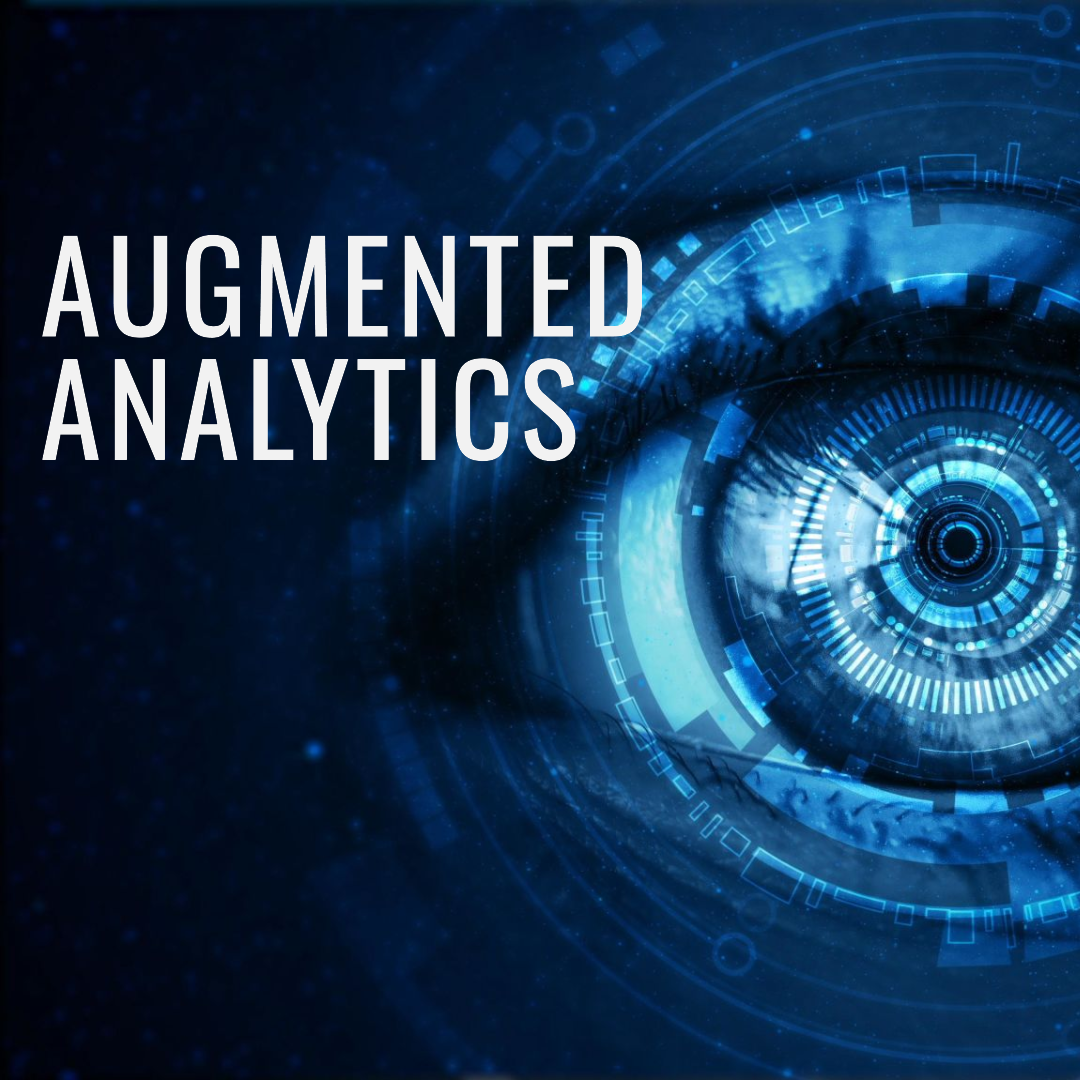
Augmented Analytics leverages AI and ML to enhance data preparation, analysis, and visualization. Consequently, Data Analytics and BI tools can analyze customer data to identify patterns and trends. Moreover, by incorporating advanced algorithms, these tools provide deeper insights. Ultimately, businesses can make more informed decisions based on these enhanced capabilities. Consequently, businesses can personalize their offerings and, therefore, improve customer satisfaction.
Unlock Real-Time Analytics
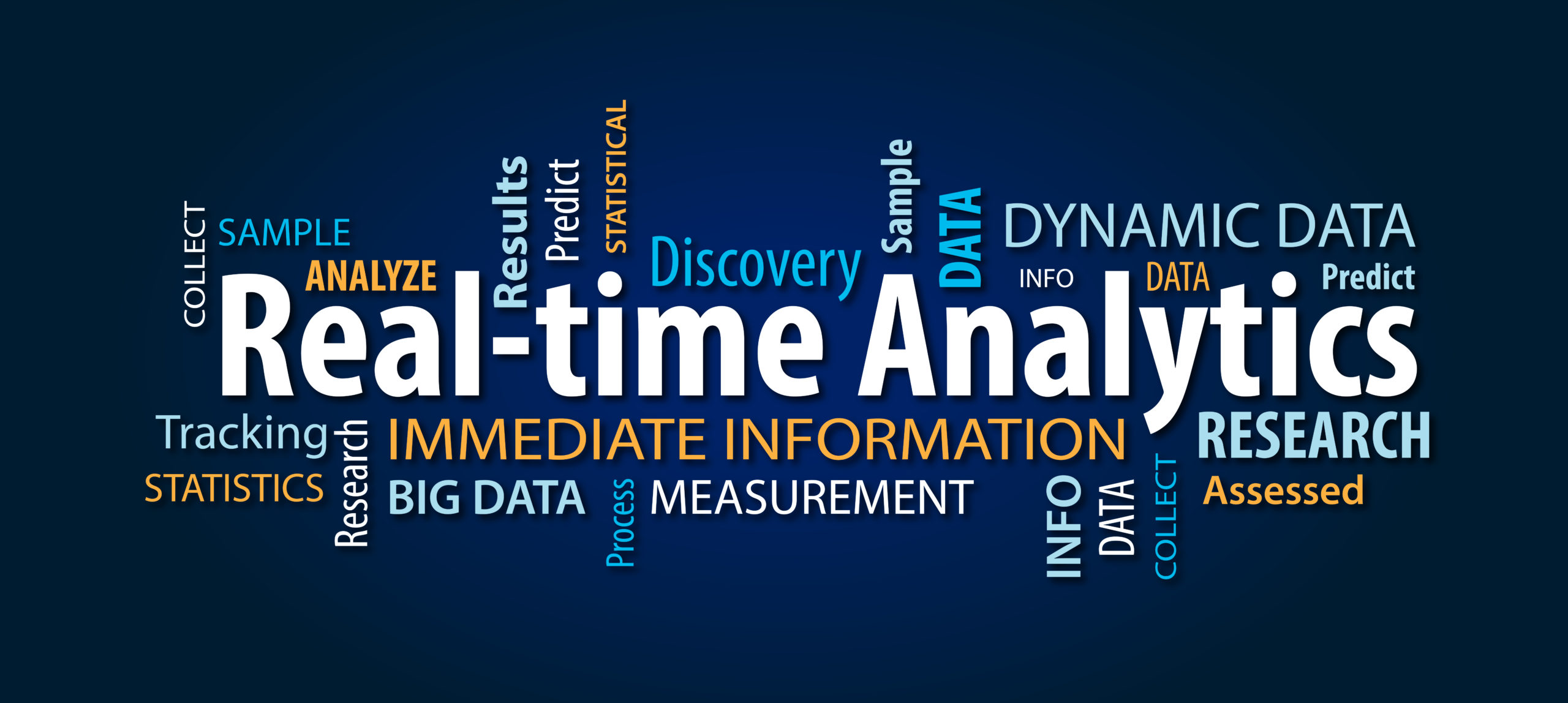
Unlock the power of Real-Time Analytics allows businesses to analyze data as it is generated, thus unlocking immediate insights and facilitating rapid decision-making. This capability is particularly valuable in industries where timely responses are critical, such as finance, healthcare, and retail.
Unlock Increased Focus on Data Governance
As data becomes increasingly central to business operations, moreover, the importance of data governance will consequently continue to grow. Furthermore, effective data governance ensures data quality, and additionally, it safeguards data security and compliance. Effective data governance ensures data quality and security; moreover, it guarantees compliance, thereby providing a solid foundation for Data Analytics and BI initiatives.
Unlock Edge Computing
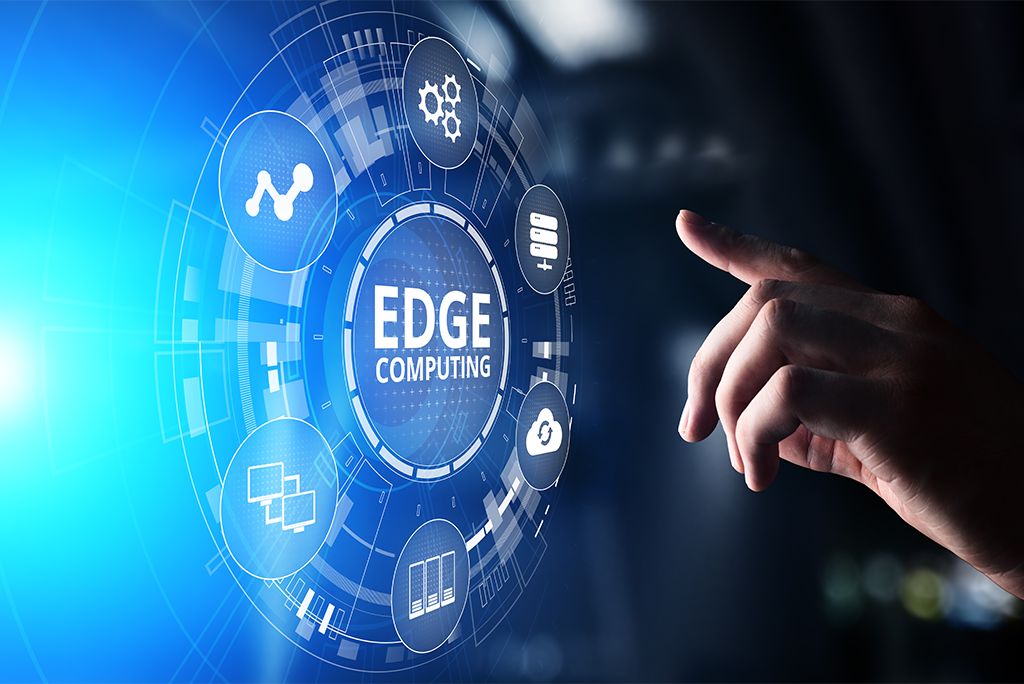
Edge Computing, on the other hand, involves processing data closer to where it is generated. Consequently, this approach reduces latency and, moreover, decreases bandwidth usage. Therefore, it enhances the efficiency and speed of data processing significantly. This is particularly relevant for IoT applications, where large volumes of data are generated at the edge of the network. Moreover, edge computing will enable more efficient data processing and, consequently, analytics in real-time.
Unlock Enhanced Data Privacy and Ethics
With growing concerns around data privacy and ethics, consequently, businesses will need to implement stronger measures to protect user data. Furthermore, they must ensure ethical practices. Additionally, implementing these measures is essential. Therefore, companies should prioritize data security and compliance. Moreover, being transparent about data usage builds trust. Ultimately, these steps will help maintain a positive reputation. This entails complying with regulations and, furthermore, being transparent about data usage, thereby ensuring data is used responsibly.
Unlock Collaborative BI
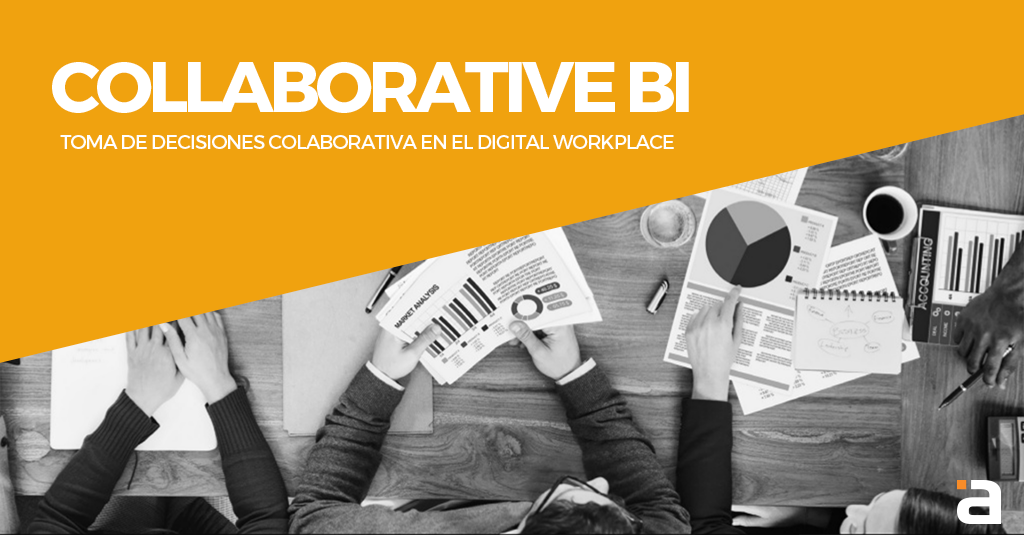
Collaborative BI, on the other hand, involves integrating BI tools with collaboration platforms, therefore facilitating better communication and decision-making among teams. Consequently, this integration enables teams to share insights and findings more effectively, ultimately leading to more informed and cohesive decisions. Consequently, this trend will enhance the ability of teams to share insights, discuss findings, and make data-driven decisions collectively. Additionally, it promotes a more collaborative approach to leveraging data within organizations.